EXPLAINABLE AI
The Responsibility Behind the Interface
By Emma Kallina
October 10, 2020
Юрий Тюленев
How Much Autonomy Would You Delegate?
Imagine you had the opportunity to pass a repetitive task (e.g. classifying images, transcribing, translating) to an autonomous system. Would you trust such an AI-based system enough to delegate agency to it? Would this change with your comprehension of the system or with different application contexts? Since a low level of trust leads to diminished use, a growing effort in research is guided towards its enhancement through increased system transparency. This research area is called Explainable AI (XAI).
Opening the Black Box?
To increase the transparency of autonomous systems, two directions have been taken in XAI:
- Explaining the Black Box: This approach aims to explain the processes underlying the system. This was shown to be successful in improving the user’s mental model of the system, for example via written explanations of the underlying processes¹, a video of the latter², or a video showing a user interacting with the system³ whilst the system’s real-time decision-making processes are simultaneously displayed.
- Leaving the Black Box Untouched: The second approach does not attempt a detailed explanation of the underlying processes but providing other additional information regarding the system. This can be in form of animation cues4 that show the systems status, the display of the certainty of the system5 about the correctness of its output, or via a proximity network visualisation6 with which users could interact to change the weight of factors underlying the algorithm.
However, both approaches have disadvantages which are addressed in the next two paragraphs.
Impractical Transparency
The idea of the first approach is undisputed: Increasing the users’ understanding of a system empowers them to make informed decisions about the trust they put into them. However, such explanations are very challenging - if not impossible - to curate in an understandable manner for more complex systems. This is critical since past research suggests that users only perceived a beneficial trade-off between invested effort and increased understanding when the explanation of the system is complete (vs. only fragmented)7. Additionally, oversimplification was even found to decrease trust.
This scrutinises the presumption that users are generally willing to invest the effort to understand a system, leading to more detailed questions: Do users differ in this willingness? Does the wish for transparency change with the application context or a growing familiarity with the system? The answers to these questions hold important implications regarding the field of XAI, changing the question from “Which is the most effective way to increase the transparency of a system?” to “How can we increase the transparency of a system in a way that matches the motivation of a specific user in a specific application context to actually review it?”. Here, less process-based - and thus less complex - explanations of the second approach could be valuable.
Intransparent Transparency
Approaches of this latter category hold great potential to empower the user. The proximity network visualisation, for example, enabled the user to assure that her priorities are reflected by the system’s algorithm, even though she might not fully understand it. Likewise, the confidence information communicates the inherent risk, thus enabling the user to make an informed decision about delegating agency.
Other strategies, however, should be regarded more carefully: Displaying the system’s status via animation cues was found to enhance the user’s trust to a level where he preferred a less accurate system over a non-animated system with better functionality. Here, trust was increased without any deeper understanding or increased control.
Careful Calibration
Such potential in influencing the user’s perception underlines the responsibility inherent to the design of the interface of autonomous systems, especially in potentially harmful application contexts (e.g. medical, transportation, military). In XAI, this is often sacrificed for a the-more-trust-the-better approach that maximises the use of autonomous systems. However, the trust that an interface evokes has to be carefully calibrated to not only match the abilities of a system, but also the application context (level of risk and costs of errors) and the personality of the user (inclination to trust or delegate control).
Considering the Human Part in the Interaction
This shifts the perspective on the user from a passive role - merely reacting on the XAI of the system - towards an active evaluation of these. This expands the field of future XAI research significantly: Under which preconditions does the user feel an increased need for transparency - and thus the motivation to review more complex explanations? Conceivable factors include the user’s personality, the moral or economic significance of the application context, and the familiarity with the system.
Such research would guide the development of highly tailored system interfaces that intuitively evoke an appropriate (not necessarily maximised) level of trust. Neither too trusting or too untrusting – but just right!
Read More
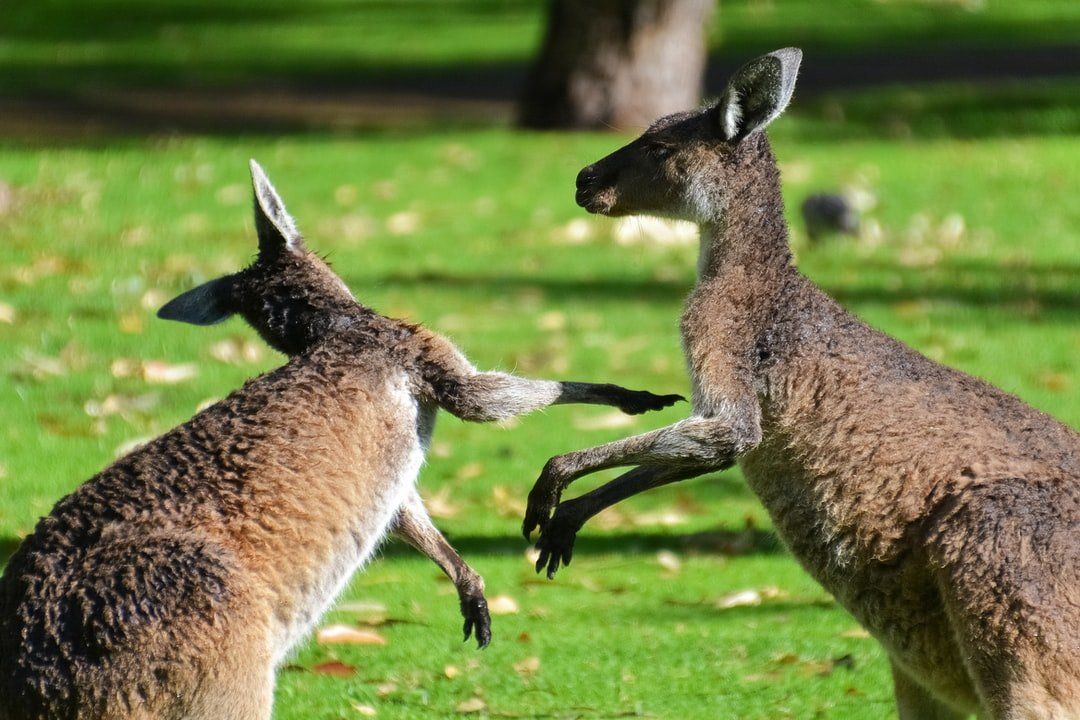
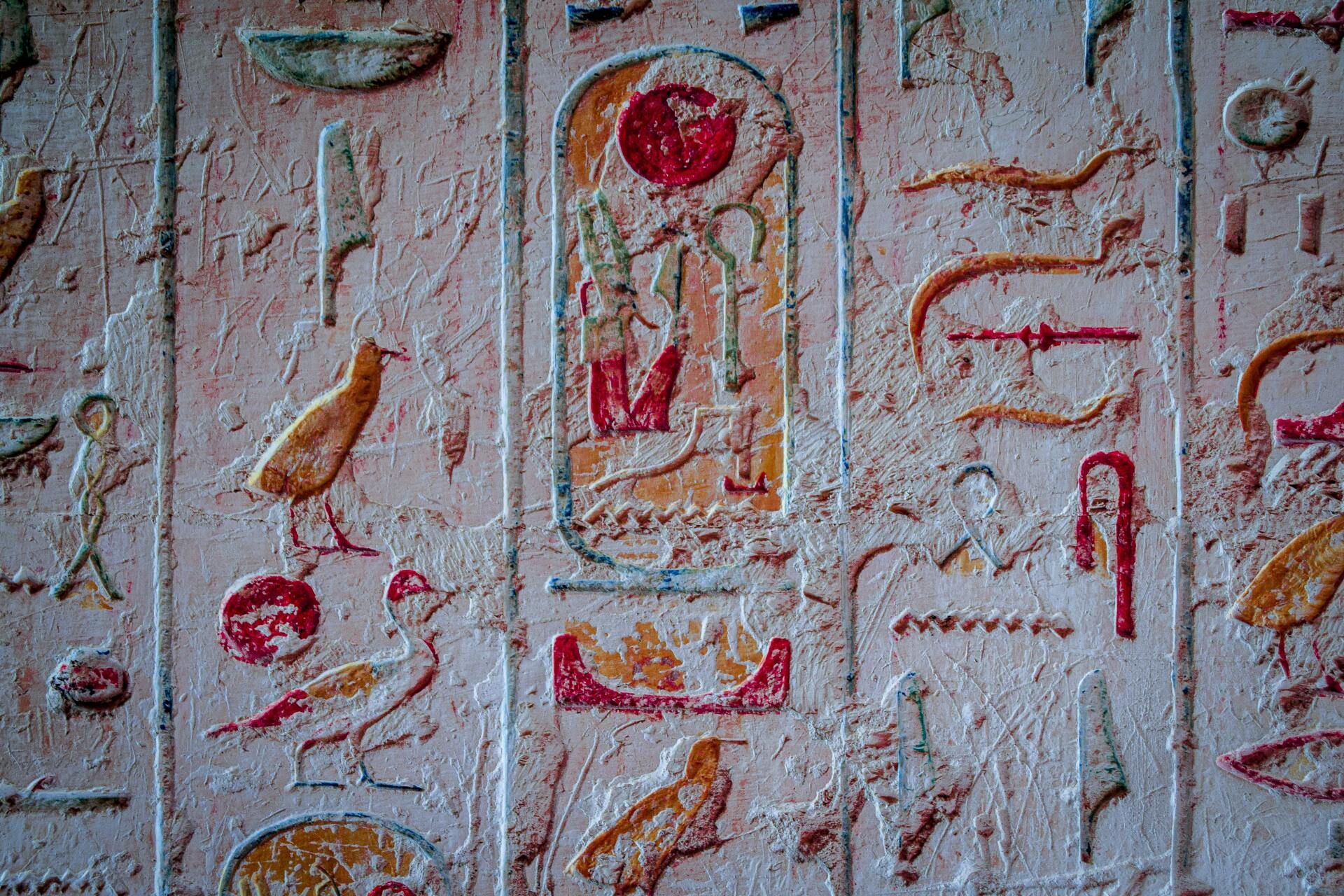
Watch Our Episodes