FAIR AI
Different Shades of Fairness in Algorithmic Decision-Making
By Mariachiara Mecati
October 10, 2020
Mohit Saini
Our daily life is deeply affected by the development and adoption of automated decision-making (ADM) systems because of the increasing tendency for humans to delegate machines to implement decision-making and output decisions or recommendations (such as credit scores, loan granting, employment screening, and various applications in the justice system), with a variety of technical approaches that range from advanced neural networks to more basic software which calculates and sorts data according to a simple set of rules.
Thus, the decisions generated by these systems play an important role on many aspects of our everyday lives: based on the collected data, for instance, a loan can be denied or a job application can be rejected, without guarantees of non-discriminatory practices.
A core element for these systems is a very broad availability of data: ADM systems are globally widespread with the aim to classify individuals and predict behaviors depending on patterns extracted from data collected about them. Consequently, the growing use of these systems gives rise simultaneously to both opportunities and risks: while opportunities often concern improved efficiency of the automated decision process, on the other side one of the main risks regards data and algorithm bias, which usually induces systemic discrimination. As biased software is a software that exposes a group (such as an ethnic minority, a type of worker or simply the gender) to an unfair treatment, an algorithm may filter and even discriminate between people under consideration, with the result of a disparate impact on different population groups.
Indeed, ADM systems include a decision-making model, an algorithm which make the model applicable as software code, input datasets used by the software, and more in general they include also the surrounding political and economic ecosystems. In this complex and extended scenario, research in Data Ethics concerns multiple aspects of the automated decision-making process and assumes an increasing relevance on many facets of the human life. Therefore, experts from several subjects are engaged in these ongoing studies, ranging from computational social scientists, data science and machine learning researchers, network engineers, as well as scholars in philosophy, law and social sciences.
Against this background, “Fairness” is a widely cited word. But what does “Fairness” stand for? Here is a simple acronym to interpret this word.
Freedom: if it is true that Fairness makes us free, it is just as true that ADM systems often work unclearly or even in unknown manner. On the contrary, precisely transparency in data and algorithms should be an essential characteristic of each automated decision-making.
Accountability: a clear responsibility for and ownership of ADM systems, with appropriate internal approving authorities as well as transparent processes and data, is a crucial point for a fair use of such systems within a firm, or an administration, or an institution, or any service that makes use of ADMs. Indeed, data subjects are encouraged to provide accurate information about themselves through several channels that aim to foster the developing digital society: at the same time, ADM systems, and particularly those that could significantly affect data subjects, need to be based on as careful understanding and knowledge of the data as possible. Alongside these two contemporary and complementary trends, accountability should focus on both internal accountability, which is concerned with the internal governance of the firm (or administration, or institutions, and so on), and external accountability, which regards the responsibility to data subjects.
Ideas and insights which are the heart of the human intelligence and, consequently, of the ongoing development of Artificial Intelligence and cutting-edge ADM systems, whose very best goals aim to facilitate and make more and more efficient decision processes and social services, as well as to improve human life in general.
Reliability regarding transparency of the trustworthiness of the system, by disclosing information about capabilities, accuracy and limitations of the system itself. What has been done to prevent, identify and mitigate discrimination and malfunctions in the system? What about possible risks, biases and impact the system can have on people? Who make a fair and conscious use of ADM systems should be able to provide answers to these important questions.
Neutrality towards people is another key point for a just society: ADM systems should be able to output neutral decisions and recommendations, without being affected by algorithm bias or flawed input data, or even by prejudice and errors in their use.
Equality, needless to say it represents a fundamental keyword of the human rights: in data science, this core aspect results in the requirement for each data subject to be Equally Represented. Indeed, discrimination carried out by ADM systems is often due to the presence of bias in the input datasets: looking back on the GIGO principle (“garbage in, garbage out”, i.e., flawed input data produces garbage as output) bias in input datasets may have propagation effects and cause biased outputs, since most of the current software-automated decisions are based on the analysis of historical data. Thus, biased datasets might lead to biased results and the need for each data subject to be Equally represented become an essential point in the developing digital society.
Society and its inherent composition should be always taken into consideration in order to treat each person equally, recognizing same social rights and providing equal social services to everybody.
Sensitive attributes are specific traits of a person (such as gender, age and race) often included in datasets used as input by ADM systems: even though anti-discrimination laws are in force in several countries and forbid unfair treatment of people based on sensitive attributes (for certain business and government services), fairness and bias in ADM systems still represent a relevant issue.
Right through this acronym we have explored the most meaningful aspects of Fairness in the complex world of automated decision-making, with a view to encouraging a more conscious and responsible use of ADM systems.
Read More
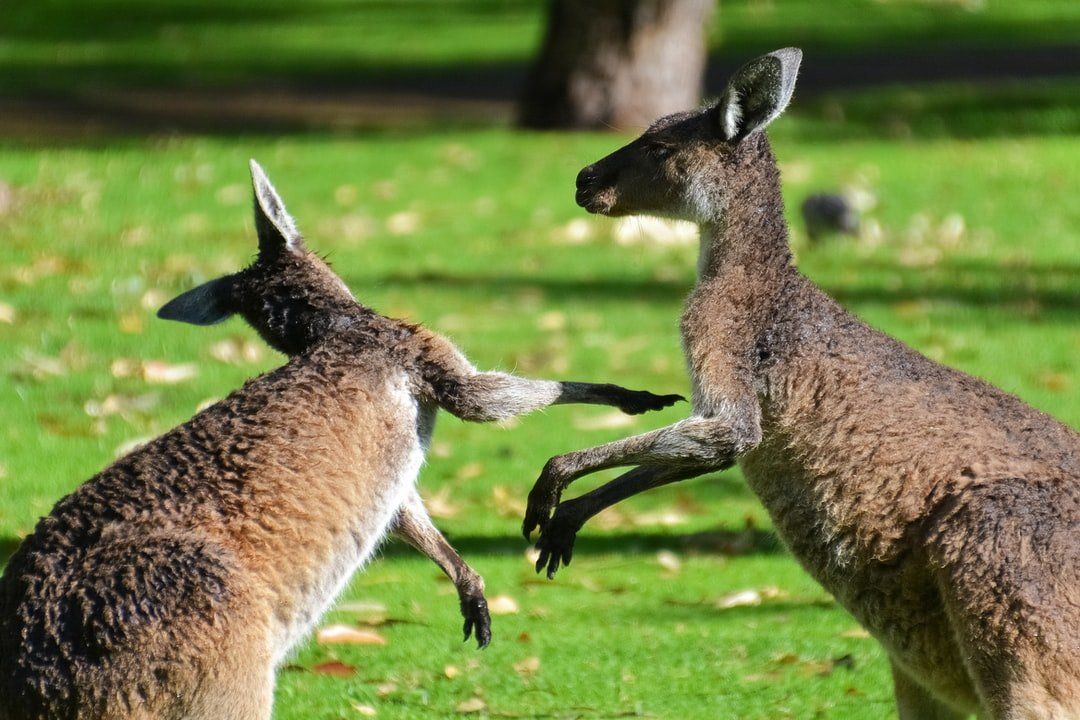
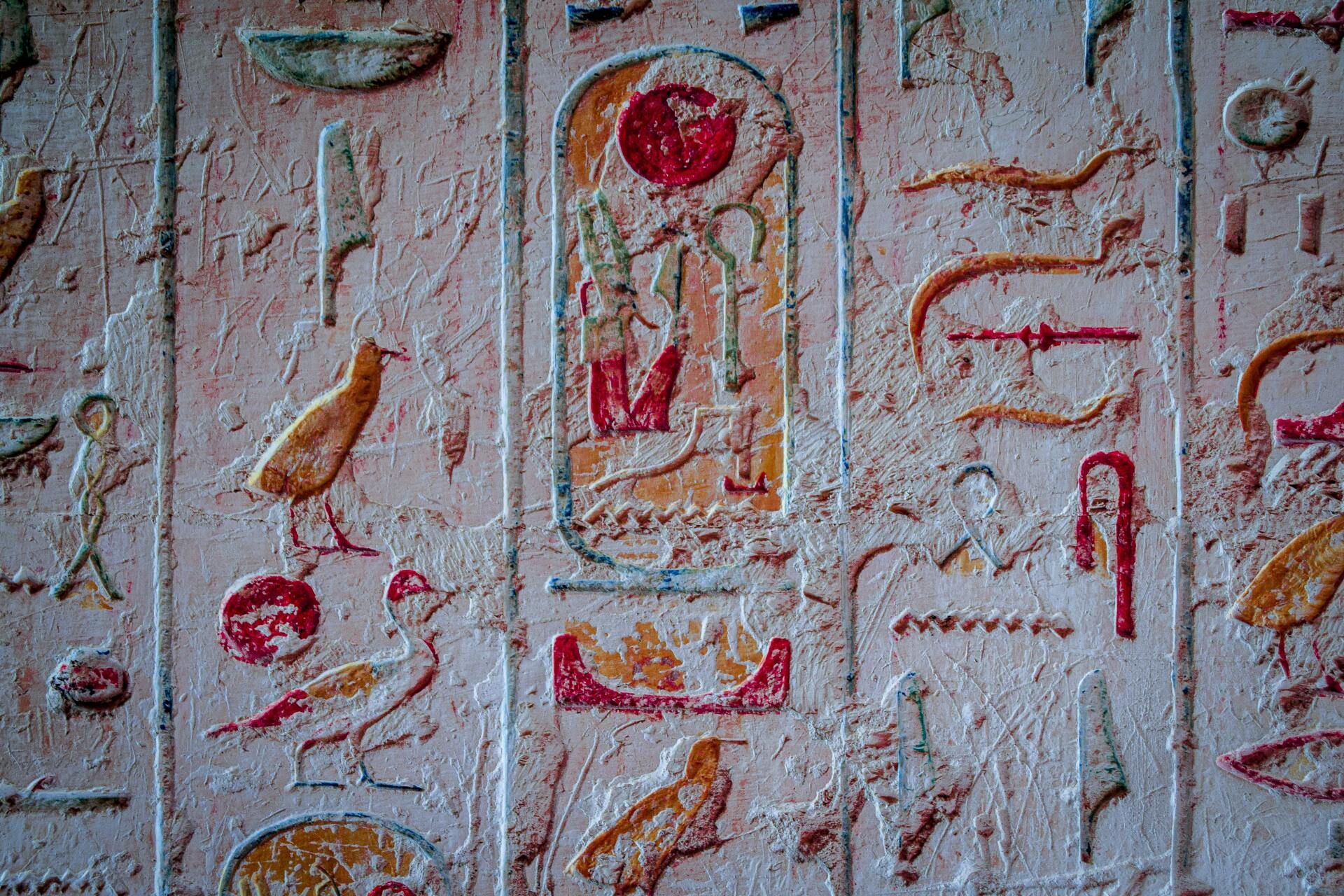
Watch Our Episodes