Over the course of our daily lives in the digital economy, we unwittingly ‘agree’ to have our data processed by a variety of entities. However, it is a universal phenomenon that it is rare for us to read terms and conditions and privacy policies and are not aware of what we agree to and what rights to our privacy we give up. Data protection legislation across the world focuses on this individualistic framework of notice-and-consent, where a user is ‘informed’ through terms of service, preventing the liability of the entity once the consent is given. These terms are always proposed to the user as an option of ‘take it or leave it’. When the only option for an individual is to either agree to the processing of their personal data for profit or to be excluded from access to the service, the power imbalance renders the consent without meaning.
In the age of aggregated data and automated algorithmic decision making, much of the information about us (be it personal or anonymized data) is collected and used without our consent for a variety of purposes, ranging from targeted advertising to predictive policing. When algorithms determine much of the information we are exposed to and the creation of concerning echo chambers, the process of how one’s price of admission affects many is abstracted. In this situation, recognizing data privacy on a collective level can help to address the existing power imbalances, and more meaningfully address discrimination in digital spaces, as well as advocate for accountability in algorithmic bias.
How can algorithms lead to discrimination?
In the system of surveillance capitalism, the processes of anonymization and aggregating data are key sources of revenue for corporations monetizing the personal data of their users. It is also a key source of data for governments, for example when using AI and ML for the purposes of contact tracing during the COVID-19 pandemic. When personal data of users is perceived as raw material for the prediction of user behaviour, one may not be aware how the submission of their data has a broader effect on a community. An individual cannot truly know how the pieces of data they have submitted is used to create patterns on a larger scale, leading to predictions and profiling about groups of people and communities.
For example, a study conducted by researchers at Northeastern University in the USA found that even without the element of human bias, the advertisement delivery algorithm of Facebook determined skewed audiences to show job ads, based on gender and race. Advertisements for nurses and cleaners among others were shown mostly to women users, and advertisements for fast food workers and cashiers among others were shown to male, African-American users. Therefore, data analytics and algorithms can define groups not only based on commonalities such as gender or religion, but on the basis of ‘ad-hoc’ categories such as consumption patterns and online behaviour. It is then reasonable to conclude for example, that based on a political viewpoint expressed or product purchased, the algorithm can create broad patterns which could in turn be used by parties like advertisers, insurers, employers and landlords to profile. This in turn has the consequence of perpetuating gender biases and discrimination, and would be further damaging for more marginalized communities, gender minorities and those from a lower socio-economic background.
How can we assert more autonomy on how our data is used for algorithms?
Scholars have argued that privacy must be seen as a public good, due to the fact that an individual’s actions or carelessness has much broader effects on society. Professor Helen Nissenbaum has argued that ‘informed consent’ is fundamentally flawed, saying, “proposals to improve and fortify notice-and-consent, such as clearer privacy policies and fairer information practices, will not overcome a fundamental flaw in the model, namely, its assumption that individuals can understand all facts relevant to true choice at the moment of pair-wise contracting between individuals and data gatherers.” When society collectively acknowledges that we rarely understand the terms on which we surrender our personal data, there cannot be any assumption of consenting to our data being used in an algorithm and the future predictions and biases that may lead to. This is further detrimental given that the adverse consequences of bias and profiling most often affect minority populations. There is simply no way that a user can meaningfully take back control in such a situation. Automated decision making has not only led to an infringement of privacy, but behavioural modification on a large scale. When a modification in a user’s behaviour is the product that is being sold and consistently tweaked to achieve the desired result for a third party corporation, the model of informed consent seems a mere band-aid solution.
It is increasingly crucial to identify ways in which we can have a say in the algorithms that have come to dominate our lives and decisions. This is where collective consent may be of use. Collective consent could bridge the gap between situations where consent can only be obtained from an individual (for example, one’s name or phone number) and governmental regulation (for instances where masses may have similar data). For example, if collectives of people could decide on a template for a privacy policy, End User License Agreement or Terms and Conditions we could reasonably agree to, there is great potential for increasing understanding about how personal data is put towards algorithms, and increasing fairness in the process of automated algorithmic decision making. If we as a collective could frame standards for the collection of quantitative and qualitative data and have surveys published, it would contribute towards removing a certain element of secrecy from the algorithms that make use of our data. The publication of such survey .results would also contribute to improving efforts in advocacy for privacy and democratic digital governance.
Democratizing algorithmic decision making: Possible ways forward
Several solutions have been posed to enable models of collective consent, such as data trusts. Data trusts are independent institutional structures, where data is placed under the stewardship of a trustee or board of trustees. Trustees would have a fiduciary responsibility to the beneficiaries of the data trust, that is, the community of people who have consented to keep their data in it. The concept promotes the beneficial use of data for purposes that are catered towards public interest. A collective under a trust would have more agency in how their data is used, collected, managed, and returned. Other data governance models include data co-operatives, data commons, and consent champions. A working example is the UK Biobank, a repository of medical data on more than 500,000 people who have consented to their data being used for research into cures, treatment and diagnosis of life threatening diseases. In the context of the COVID-19 pandemic and concerns regarding the inadequate privacy protections provided by contact tracing applications, the data trust model could have significant applications.
There remain pertinent questions with respect to collective consent- the composition of collectives will defer based on the context, the fiduciary duties of a trust or steward would have to be clearly defined, and the modalities of enforcement must be determined, especially if the collective or community consists of vulnerable and marginalized populations. However, it is a step forward in re-conceptualizing data as a commons, and the internet as a public utility. An interesting offshoot of a requirement for collective consent could also be that the technology industry and other industries (such as health) could also engage in wider information campaigns to justify the benefits of the collection and use of personal data. A recent update to Google’s application in smartphones is a page called ‘your data in search’, which justifies why user data is important to improve the service.
While such campaigns may be one sided explanations, the possibility of increasing transparency and reducing the secrecy commonly associated with algorithms has the potential to democratize our understanding of how predictions are made, what we are willing to agree to, and where we draw the line when it comes to automated decision making, bias and harmful information bubbles. Consent can truly be informed when we are able to understand how in the context of algorithmic decision making, the actions of one person can affect us all.
Read More
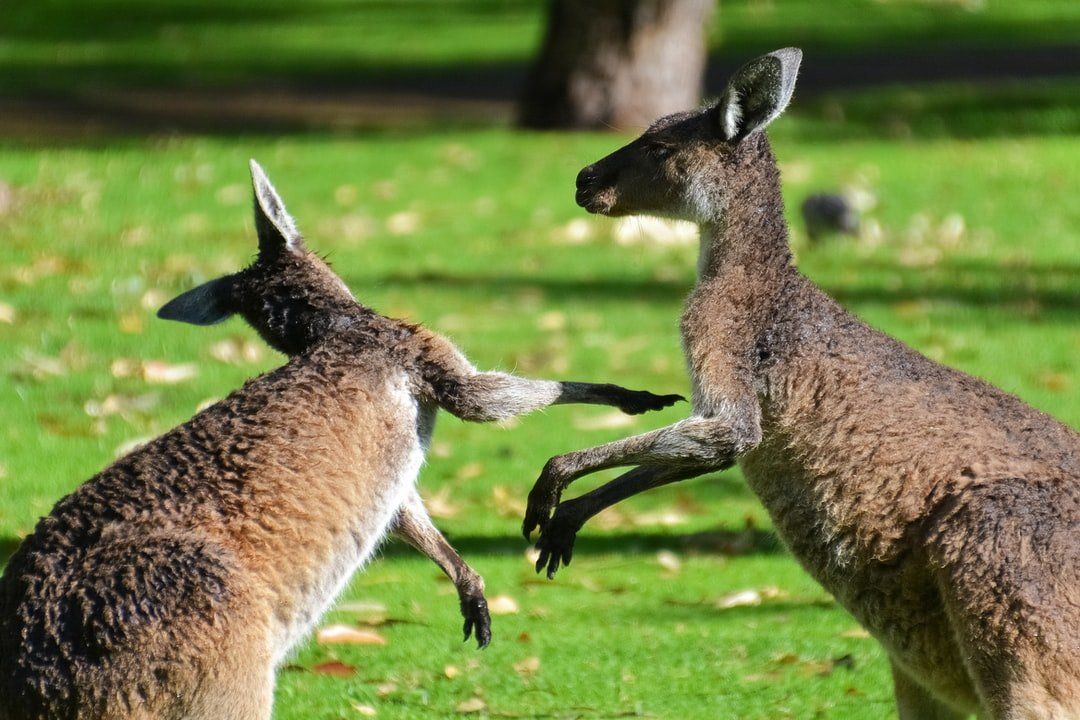
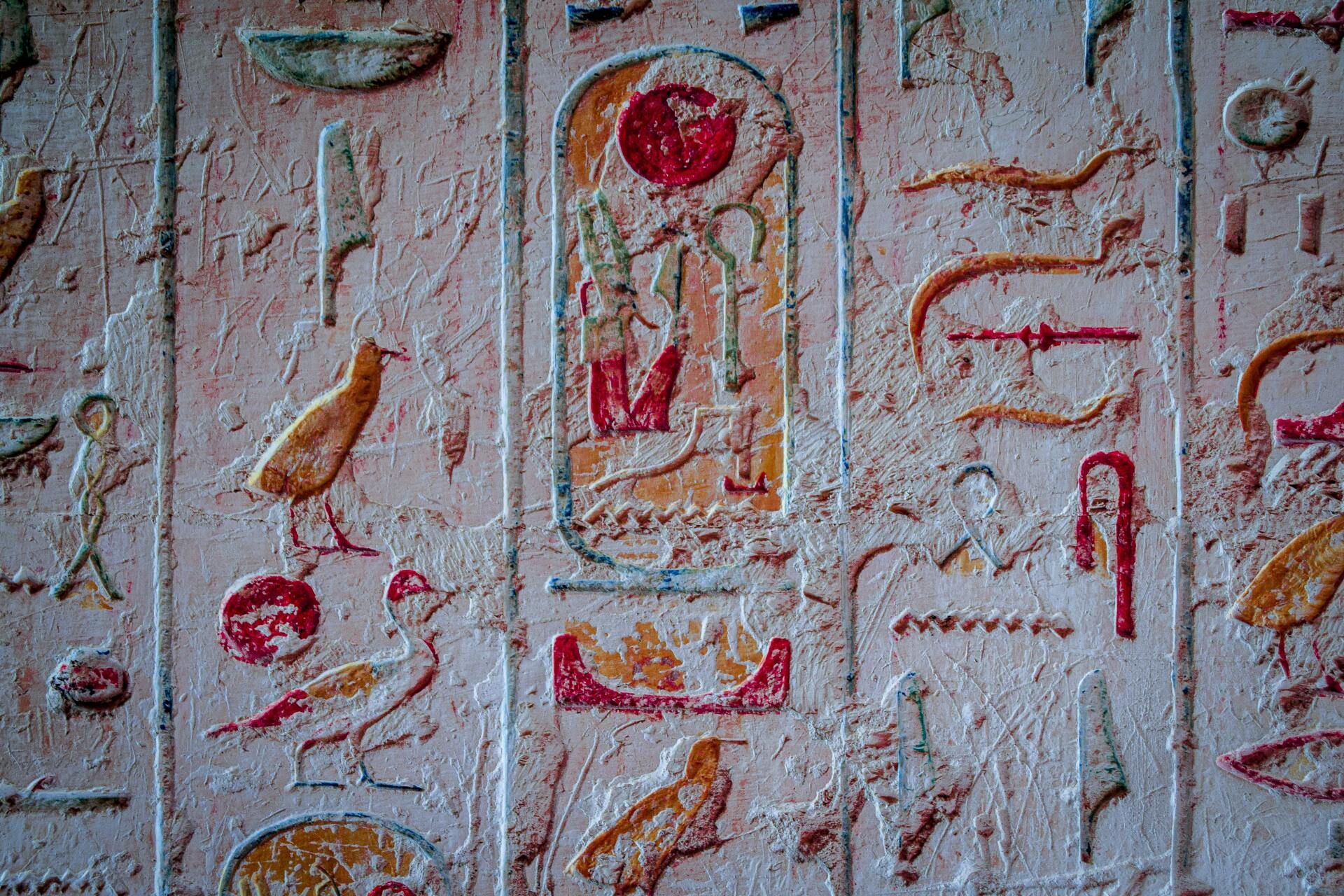
Watch Our Episodes