AI DISCRIMINATION
Can I Get A Loan?
By Elena Beretta
August 30, 2020
John is a divorced father who has two dependent children living with him. In the morning he turns off the alarm clock, gets up, and first reads the news on Facebook while listening to the music recommended by Spotify. He takes his children to school and then enters on Google Maps the address of his bank, where he needs to ask for a loan. John never remembers the exact address because he lives far away in a popular and suburban area of the city. Once he arrives, he fills in a loan application form.
What is your gender?
Male
What is your ethnicity?
Caucasian
Are you married?
No
Do you have any dependent children?
Yes
Do you live in a suburban area?
Yes
After two days, an automatic response from the bank notifies him that the loan application has been declined.
"We regret to inform you that because of your gender, the neighborhood you live in and your ethnicity, you are not eligible to receive the loan".
As you can easily imagine, this is a fictional story. No human being or automatic decision-making system will discriminate against John because he is Caucasian or because he is a man, and yet this story has a kernel of truth.
We live in a historical era in which machine learning and artificial intelligence systems (ML&AI) are increasingly widespread in our economies (Pasquale 2015). These systems make growing and massive use of a large amount of data on human behavior collected through various channels (e.g. social media, apps, telephone data, credit card transactions, search engines, etc.). The extensive and large-scale use of this data for different purposes is rapidly transforming different areas of our daily lives. ML&AI systems are in fact increasingly involved in shaping decisions that have a significant impact on individual and collective life as well as affecting fundamental public functions such as the administration of justice; primary public interests such as security, crime prevention, counter-terrorism, or control of migration flows; essential public services such as the education system or access to other social services. In all these areas, ML&AI systems show extremely productive results, especially in terms of greater efficiency and rapidity of decision-making processes - unlike human beings, ML&AI systems do not get tired or bored -.
However, the opposite is also true. In fact, ML&AI systems have the potential not only to make mistakes, but also to take - without adequate corrective action - highly discriminatory decisions. Several studies have in fact highlighted some relevant issues related to these systems which in some cases, when affected by distortions, systematically and unfairly discriminate against certain individuals or groups in favor of others, denying opportunities or generating undesirable results for inappropriate reasons, as in John's case.
The way machine learning systems operate is a crucial issue for our societies (O'Neal 2016). Algorithms are designed to recognize winners and losers, specifying precisely which situations lead to satisfactory results; they are shaped to look for patterns and characteristics in individuals who have historically led to success, not by making things right when used randomly, but by replicating past patterns and practices.
A relevant example is the recent case presented by the ProPublica survey site on COMPAS recidivism tool, an algorithm employed by the judicial American system to inform criminal convictions in predicting the risk of recidivism. In particular, the study found that the COMPAS was more likely to labeling black defendants at higher risk compared to white defendants - twice as high as false positives and half of false negatives -.
In the last few years, several solutions and formal definitions of equity have been suggested by the machine learning community in order to mitigate the discriminatory effect of ML&AI systems (Barocas 2018). However, the solutions proposed mostly focus on mathematical aspects of the models. Researches pointing out the need to implement socio-technical systems in which fairness is treated not merely as strictly technical but also as a social component, are still very few (Beretta 2019). Although research is increasingly focusing on finding solutions to mitigate the segregation effect of ML&AI systems, many efforts still need to be made in order to understand when a system fails, why it fails and what are the social costs of failure (Corbett 2017).
Therefore, designing an ML&AI fair system means firstly to assess the implications of choosing one type of fairness instead of another in a certain social context; secondly, to assess the degree of acceptability depending on the context and the chosen fairness criterion. Taking into account that it is not possible to implement more than one type of fairness simultaneously (Kleinberg 2016), the design choices have an extremely relevant impact on the effect that the system’s results will have on society. The integration of social values and democratic assumptions embedded in the current mathematical formalizations of fairness is therefore crucial.
The debate on fairness in the field of machine learning shows a profound and relatively worrying lack: although researchers are reacting in a positive and proactive way, data scientists and computer engineers are increasingly involved in making decisions that affect individuals by acting as judges in decision-making processes, although society as a whole should assume this role.
References
Barocas, S.; Hardt, M.; and Narayanan, A. 2018. Fairness and Machine Learning. fairmlbook.org. http://www.fairmlbook.org
Beretta, E., Santangelo, A., Lepri, B., Vetr ́o, & De Martin, J. C. 2019. The Invisible Power of Fairness. How Machine Learning Shapes Democracy. In Meurs, M.-J., &Rudzicz, F. (Eds.),Advances in Artificial Intelligence, Proceedings of 32nd CanadianConference on Artificial Intelligence, Canadian AI 2019, Vol. 11489, pp. 238–250.Springer, Cham.
Corbett-Davies, S.; Pierson, E.; Feller, A.; Goel, S.; and Huq, A. 2017. Algorithmic decision making and the cost of fairness. In Proceedings of the 23rd ACM SIGKDD International Conference on Knowledge Discovery and DataMining (KDD 2017).
O’Neil, C. 2016. Weapons of Math Destruction: How Big Data Increases Inequality and Threatens Democracy. NewYork, NY: Crown Publishing Group.
Pasquale, F. 2015. The Black Box Society: The Secret Algorithms That Control Money and Information. Cambridge, MA, USA: Harvard University Press.
Read More
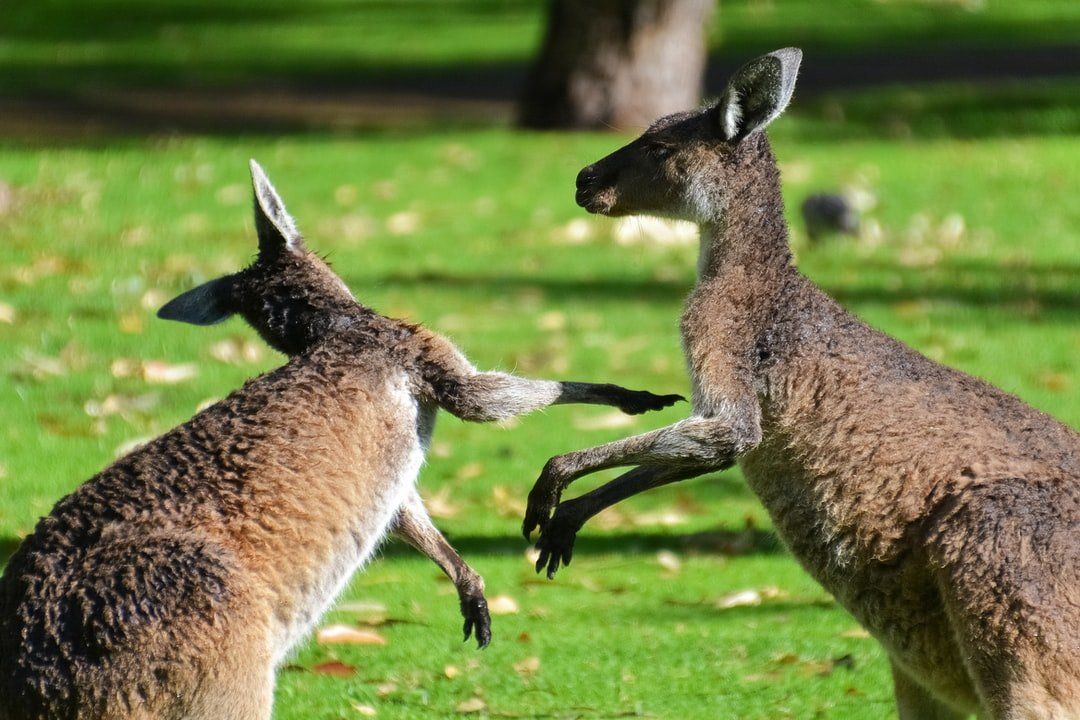
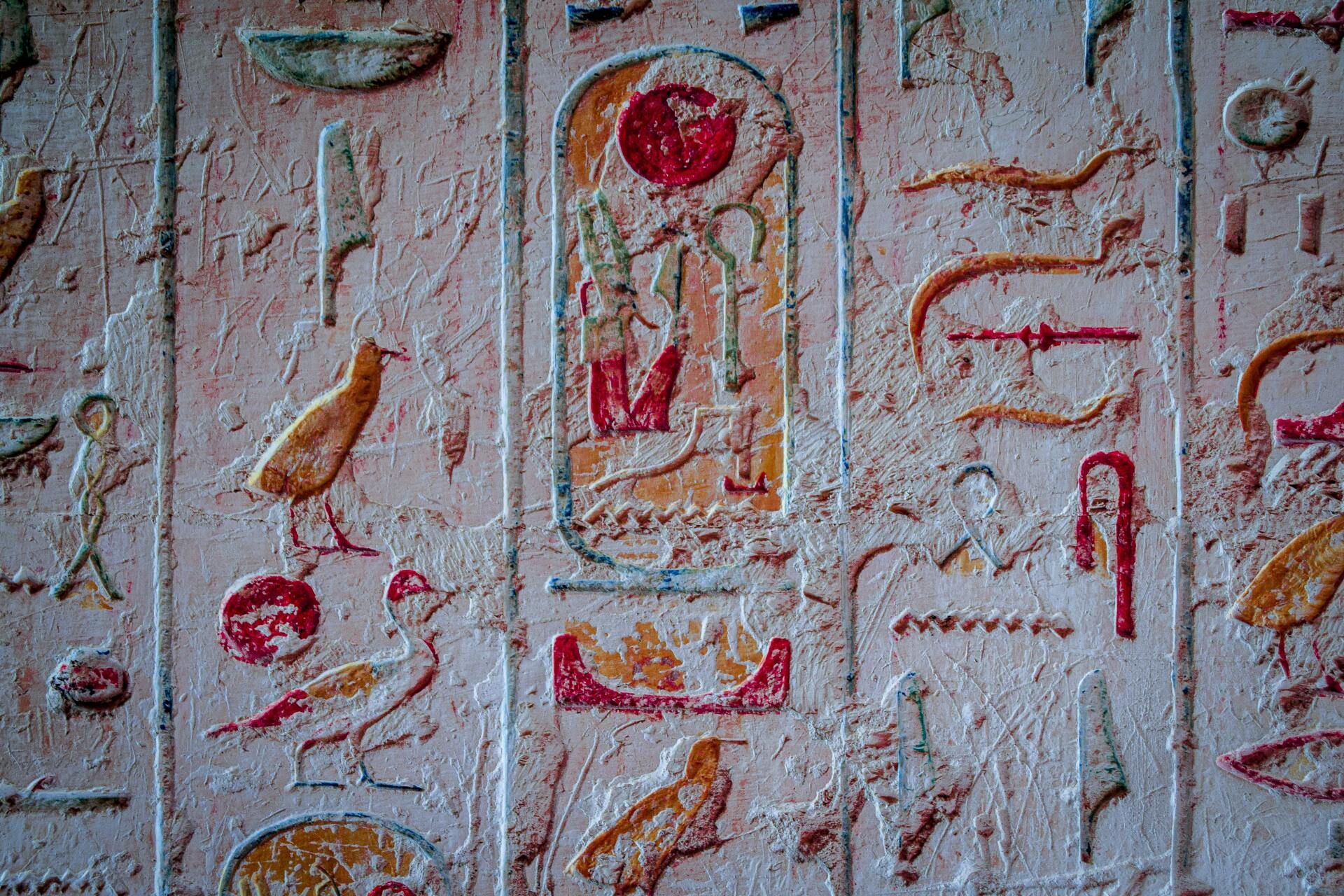
Watch Our Episodes